Aaron Klein
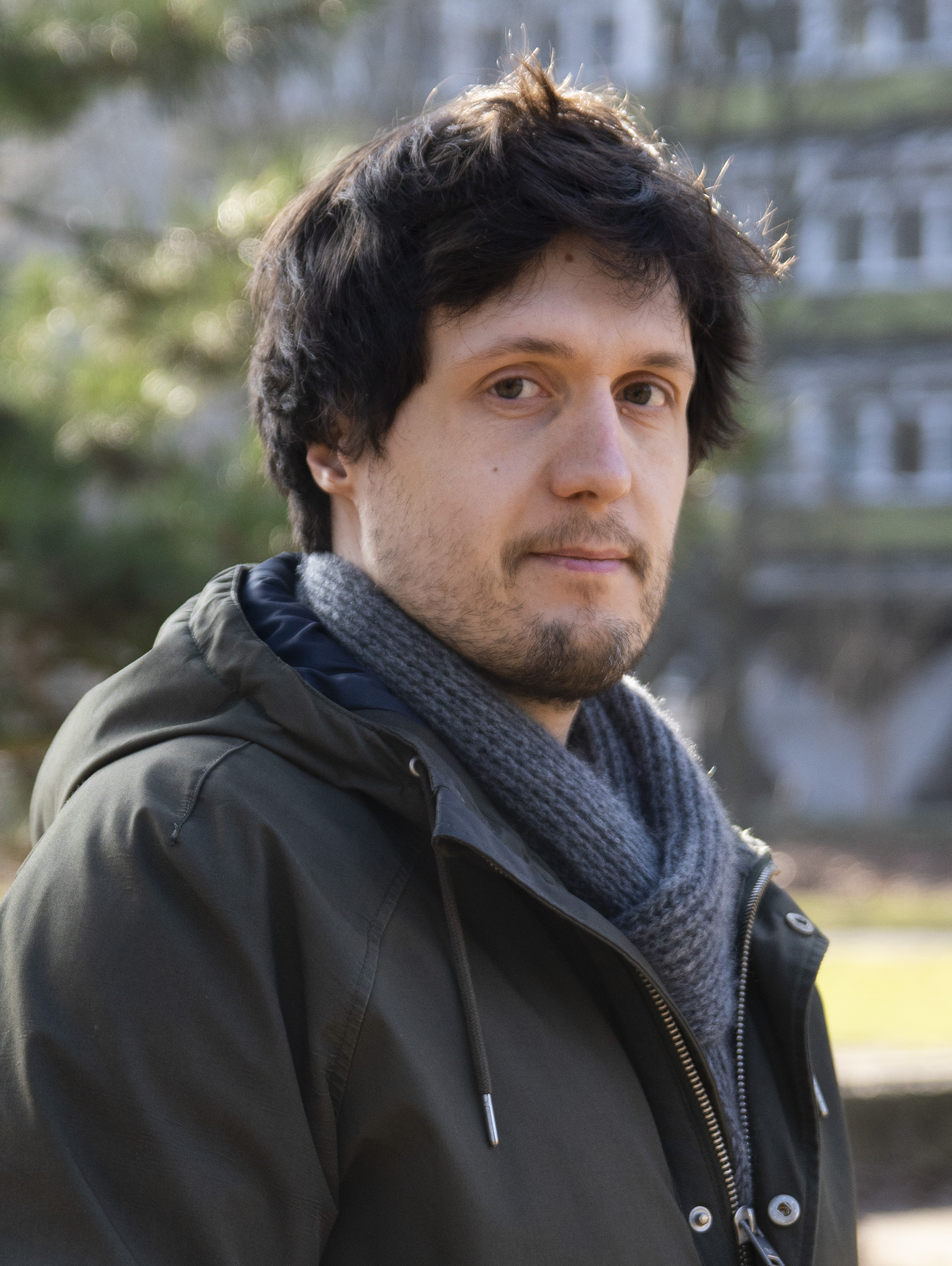
The goal of my research is to develop machine learning approaches that can configure themselves automatically by learning from past data. In the long term, I hope that my work will contribute to fulfilling the promise of artificial intelligence and lead to complete end-to-end learning systems.
More specifically, my research interests include:
- Automated machine learning
- Neural architecture search
- Bayesian hyperparameter optimization
- Model compression of large language models
- Automated prompt engineering
short bio
I am the head of the AutoML research group at ScaDS.AI (Center for Scalable Data Analytics and Artificial Intelligence) in Leipzig. I am also a co-host of the virtual AutoML Seminar as part of the ELLIS units in Berlin and Freiburg. Alongside my collaborators, I lead the development of the open-source library SyneTune for large-scale hyperparameter optimization and neural architecture search.
Until 2024, I worked as an applied scientist at AWS, where I was part of the long-term science team of SageMaker, AWS’s machine learning cloud platform, and the science team of Amazon Q, the GenAI assistant of AWS. Prior to that, I finished my PhD at the University of Freiburg under the supervision of Frank Hutter in 2019. In 2022, my co-authors and I won the best paper award at the AutoML Conference. My collaborators from the University of Freiburg and I won the ChaLearn AutoML Challenge in 2015. I co-organized the workshop on neural architecture search at ICRL 2020 and ICLR 2021, respectively and served as local chair for the AutoML Conference in 2023. I also regularly serve as a reviewer for ICML, ICLR, NeurIPS, TMLR, and JMLR.
news
May 31, 2025 | One ICML paper and one AutoML Conf paper accepted. |
---|---|
Apr 06, 2025 | Together with Pascal Kerschke, I am looking for a PhD Student to work on multi-objective Neural Architecture Search. Find more information here |
Apr 01, 2025 | I will give a talk at the AutoML Summer School 2025 |
Sep 26, 2024 | Our paper on HW-GPT-Bench: Hardware-Aware Architecture Benchmark for Language Models got accepted at the NeurIPS 2024 DBT Track. |
Aug 06, 2024 | Our paper on Structural Pruning of Pre-trained Language Models via Neural Architecture Search got accepted at TMLR. |